Case Studies
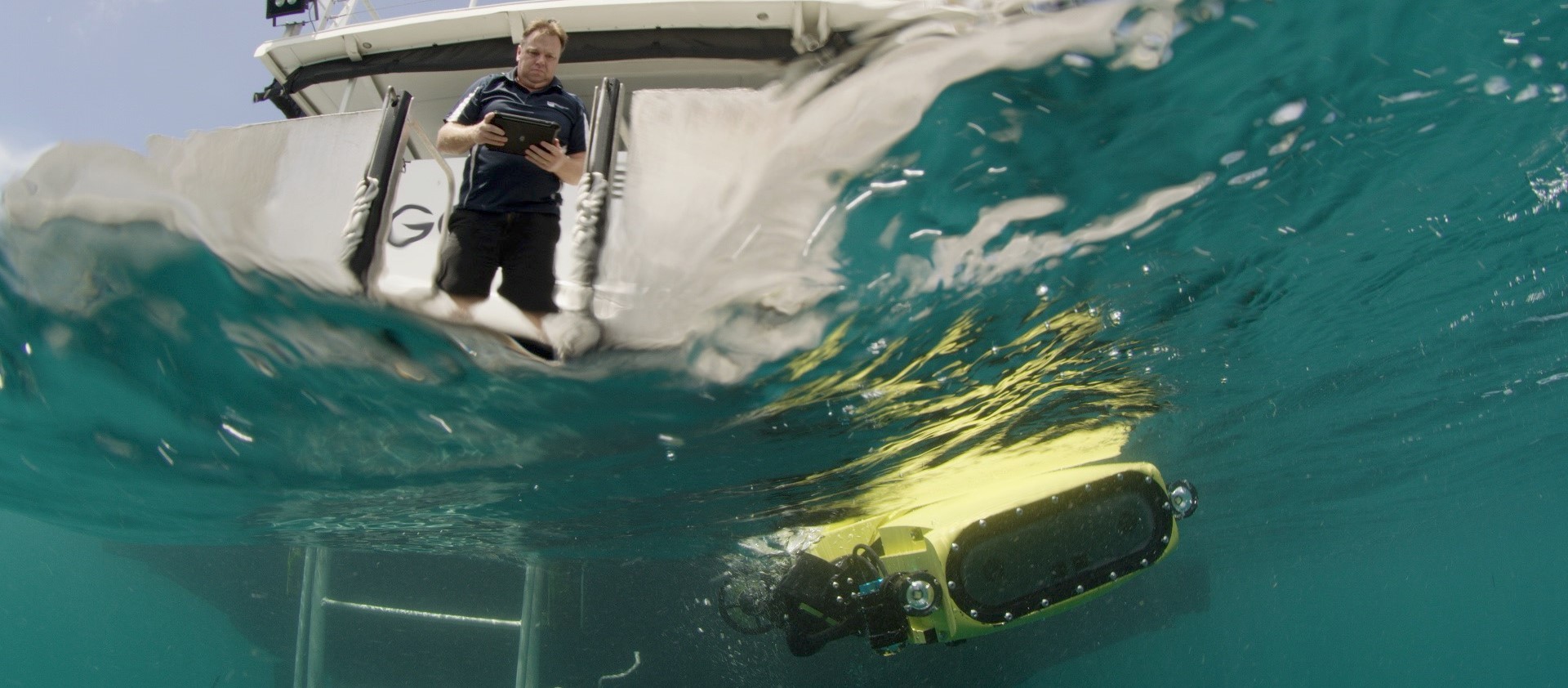
Our ambitious research programs are helping to create robots that see and understand for the sustainable wellbeing of people and the environment in which they live.
Future Surgical SnakeBots Push the Boundaries of Evolution in a Battle for Human Good
$2 Million High Five!
Back to the Future! The Birth of DDNs
Prescribing a Social Robot ‘Intervention’
AI Reaches New Heights
How Do Robots ‘Find’ Themselves?
Flying Robots to the Rescue
Connected and Highly Automated Driving (CHAD) Pilot
Embracing Chaos: VSLAM 101 for Real-World Robots on the Move!
Could this be the World’s Most Decorated Marine Robot?
Farm-Hand Robots
Robotic Vision and Mining Automation
What do Flying Robots and Star Wars Speeder Bikes have in Common?
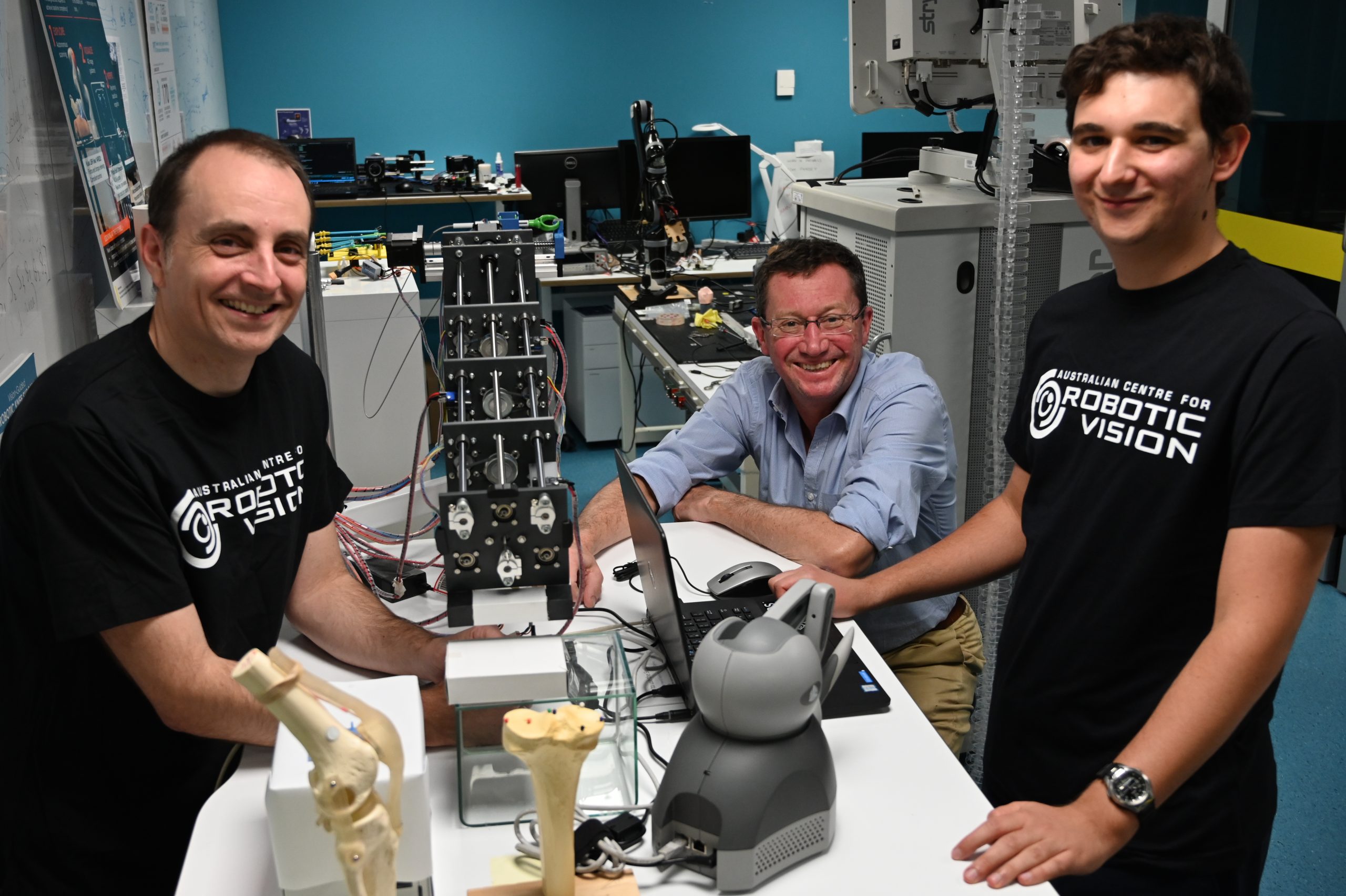
Future Surgical SnakeBots Push the Boundaries of Evolution in a Battle for Human Good
In a world first, Centre researchers are pushing the boundaries of evolution to create bespoke, miniaturised surgical robots, uniquely matched to individual patient anatomy.
The cutting-edge research project is the brainchild of PhD Researcher Andrew Razjigaev, who, in 2018, developed the Centre’s first SnakeBot prototype designed for knee arthroscopy.
In 2019, the researcher, backed by the Centre’s world-leading Medical and Healthcare Robotics Group, has taken nothing short of a quantum leap in the surgical robot’s design. In place of a single robot, the new plan envisages multiple snake-like robots attached to a RAVEN II surgical robotic research platform, all working together to improve patient outcomes.
The genius of the project extends to development of an evolutionary computational design algorithm that creates one-of-a-kind, patient-specific SnakeBots in a ‘survival-of-the-fittest’ battle.
Only the most optimal design survives. That is, the one best suited to fit, flexibly manoeuvre, and see inside a patient’s knee, doubling as a surgeon’s eyes and tools, with the added bonus of being low-cost (3D printed) and disposable.
Centre Chief Investigator Jonathan Roberts and Associate Investigator Ross Crawford, who jointly lead the QUT-based Medical and Healthcare Robotics Group, believe the semi-autonomous surgical system could revolutionise keyhole surgery in ways not before imagined.
The aim of the robotic system is to assist, not replace surgeons, ultimately improving patient outcomes.
Due to complete his PhD research project by early 2021, Andrew Razjigaev graduated as a mechatronics engineer at QUT in 2017 and has been a part of the Centre’s Medical and Healthcare Robotics Group since 2016.
“Robotics is all about helping people in some way and what I’m most excited about is that this project may lead to improved health outcomes, fewer complications and faster patient recovery,” he said.
“That’s what really drives my research – being able to help people and make a positive difference. Knee arthroscopy is one of most common orthopaedic procedures in the world, with around four million procedures a year, so this project could have a huge impact.”
Did you know? Escalating use of robots and robotic technology in the healthcare space is a global trend. Its adoption is seen as a way of increasing the quality of healthcare, improving recovery time and reducing the need for further medical intervention. Importantly, development of robotics in medicine relies on the fusion of teams from different science and engineering backgrounds. The Medical and Healthcare Robotics Group at the Centre is comprised of engineers, scientists, biologists and industrial designers – all working together to solve very complex problems. The group is co-led by Chief Investigator Jonathan Roberts and Associate Investigator Ross Crawford, who is also an orthopaedic surgeon.
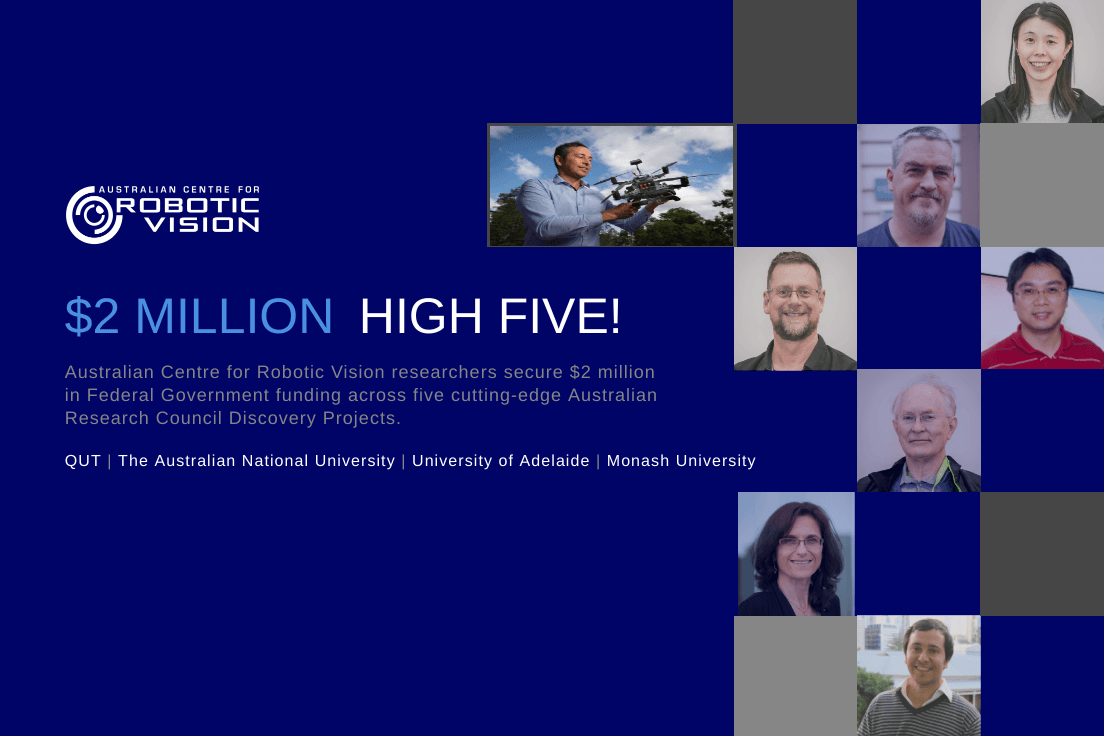
$2 Million High Five!
When every second counts: Multi-drone navigation in GPS-denied environments ($360,000 / QUT)
Associate Professor Felipe Gonzalez; Associate Professor Jonghyuk Kim; Professor Sven Koenig; Professor Kevin Gaston
The aim of this research is to develop a framework for multiple Unmanned Aerial Vehicles (UAV), that balances information sharing, exploration, localization, mapping, and other planning objectives thus allowing a team of UAVs to navigate in complex environments in time critical situations. This project expects to generate new knowledge in UAV navigation using an innovative approach by combining Simultaneous Localization and Mapping (SLAM) algorithms with Partially Observable Markov Decision Processes (POMDP) and Deep Reinforcement learning. This should provide significant benefits, such as more responsive search and rescue inside collapsed buildings or underground mines, as well as fast target detection and mapping under the tree canopy.
Active Visual Navigation in an Unexplored Environment ($450,000 / University of Adelaide)
Professor Ian Reid; Dr Seyed Hamid Rezatofighi
This project will develop a new method for robotic navigation in which goals can be specified at a much higher level of abstraction than has previously been possible. This will be achieved using deep learning to make informed predictions about a scene layout, and navigating as an active observer in which the predictions informs actions. The outcome will be robotic agents capable of effective and efficient navigation and operation in previously unseen environments, and the ability to control such agents with more human-like instructions. Such capabilities are desirable, and in some cases essential, for autonomous robots in a variety of important application areas including automated warehousing and high-level control of autonomous vehicles.
Deep Learning that Scales ($390,000 / University of Adelaide)
Professor Chunhua Shen
Deep learning has dramatically improved the accuracy of a breathtaking variety of tasks in AI such as image understanding and natural language processing. This project addresses fundamental bottlenecks when attempting to develop deep learning applications at scale. First, this project proposes efficient neural architecture search that is orders of magnitude faster than previously reported, abstracting away the most complex part of deep learning. Second, we will design very efficient binary networks, enabling large-scale deployment of deep learning to mobile devices. Thus this project will overcome two primary limitations of deep learning generally, however, and will greatly increase its already impressive domain of practical application.
3D Vision Geometric Optimisation in Deep Learning ($390,000 / The Australian National University)
Professor Richard Hartley; Dr Miaomiao Liu
This project aims to develop a methodology for integrating the algorithms of 3D Vision Geometry and Optimization into the framework of Machine Learning and demonstrate the wide applicability of the new methods on a variety of challenging fundamental problems in Computer Vision. These include 3D geometric scene understanding, and estimation and prediction of human 2D/3D pose and activity. Applications of this technology are to be found in Intelligent Transportation, Environment Monitoring, and Augmented Reality, applicable in smart-city planning and medical applications such as computer-enhanced surgery. The goal is to build Australia’s competitive advantage in the forefront of ICT research and technology innovation.
Advancing Human–robot Interaction with Augmented Reality ($360,000 / Monash University)
Professor Elizabeth Croft; Professor Tom Drummond; Professor Hendrik F. Van der Loos
This research aims to advance emerging human-robot interaction (HRI) methods, creating novel and innovative, human-in-the-loop communication, collaboration, and teaching methods. The project expects to support the creation of new applications for the growing wave of assistive robotic platforms emerging in the market and de-risk the integration of collaborative robotics into industrial production. Expected outcomes include methods and tools developed to allow smart leveraging of the different capacities of humans and robots. This should provide significant benefits allowing manufacturers to capitalize on the high skill level of Australian workers and bring more complex high-value manufactured products to market.
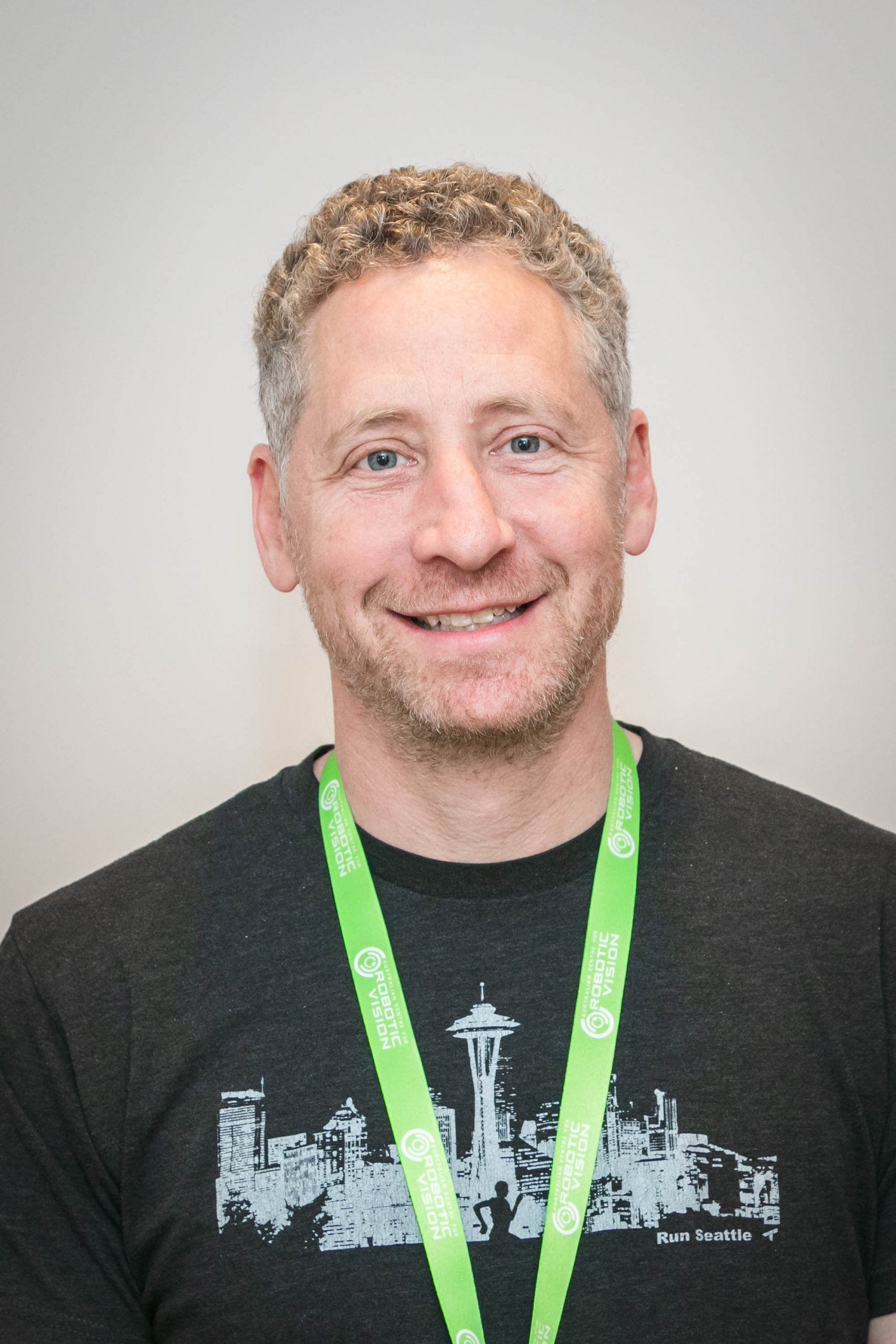
Back to the Future! The Birth of DDNs
Despite dating back to the 60s and somewhat finding its groove in the 80s, deep learning still didn’t really ‘boom’ until 2012. Hailed as a revolution in artificial intelligence, it opened the door for robots to learn more like humans via neural networks built to mimic the behaviour of the human brain.
At the dawn of a new decade, Centre researchers are deliberately taking one step back and two steps forward with the development of an entirely new and exciting class of end-to-end learnable models called deep declarative networks (DDNs).
Centre Chief Investigator Stephen Gould, who heads up our Australian National University node, believes DDNs are ‘the next big thing’. He sees DDNs as offering a new hope to robotics and computer vision communities who ‘lost something in adopting deep learning over traditional computer vision models’.
Promising the best of both worlds, DDNs incorporate mathematical and physical models developed in robotics and computer vision before 2012 and puts them inside deep learning models.
That’s one step back and two steps forward, according to Professor Gould and his colleagues, Research Fellow Dylan Campbell and Chief Investigator Richard Hartley. The trio are organising a DDN workshop at the world’s premier annual computer vision gathering, the Conference on Computer Vision and Pattern Recognition (CVPR 2020).
“Robots need to perceive (see), think (plan) and act (move and manipulate),” said Professor Gould.
“Neural networks or deep learning models have demonstrated themselves to be very powerful for the visual perception part, but getting them to combine with physical and mathematical models that are needed for the thinking and acting part, on real robots, has been hard.
“DDNs bring us a step closer in allowing the power of neural networks for visual (and language) perception to be combined with models of the world that lead to planning and action.”
Professor Gould and his team are working on how to impose hard constraints on the output of DDNs, with real-world impact on development of self-driving cars.
“Currently there’s nothing in deep learning models that enforces hard constraints,” he said.
“So, for example, in an autonomous driving scenario you might want to estimate the location of all cars around you by fitting a 3D model to what you observe.
“With current deep learning models there’s no way to say that two cars cannot occupy the same physical space or that a car must be supported by the road. As such, the model may detect two cars when there is only really one, misinterpret the location of a car, or think that a picture of a car on a billboard is a real car.
“With DDNs, we can add those sort of constraints. That doesn’t necessarily guarantee that the model gets everything right, but at least we can remove some mistakes when we know they violate the physical dimensions of the real world.”
Want to find out more? Professor Gould takes a deep dive in this LinkedIn article, explaining why DDNs are more robust and interpretive than conventional deep learning models, including an ability to solve inner optimisation problems ‘on the fly’.
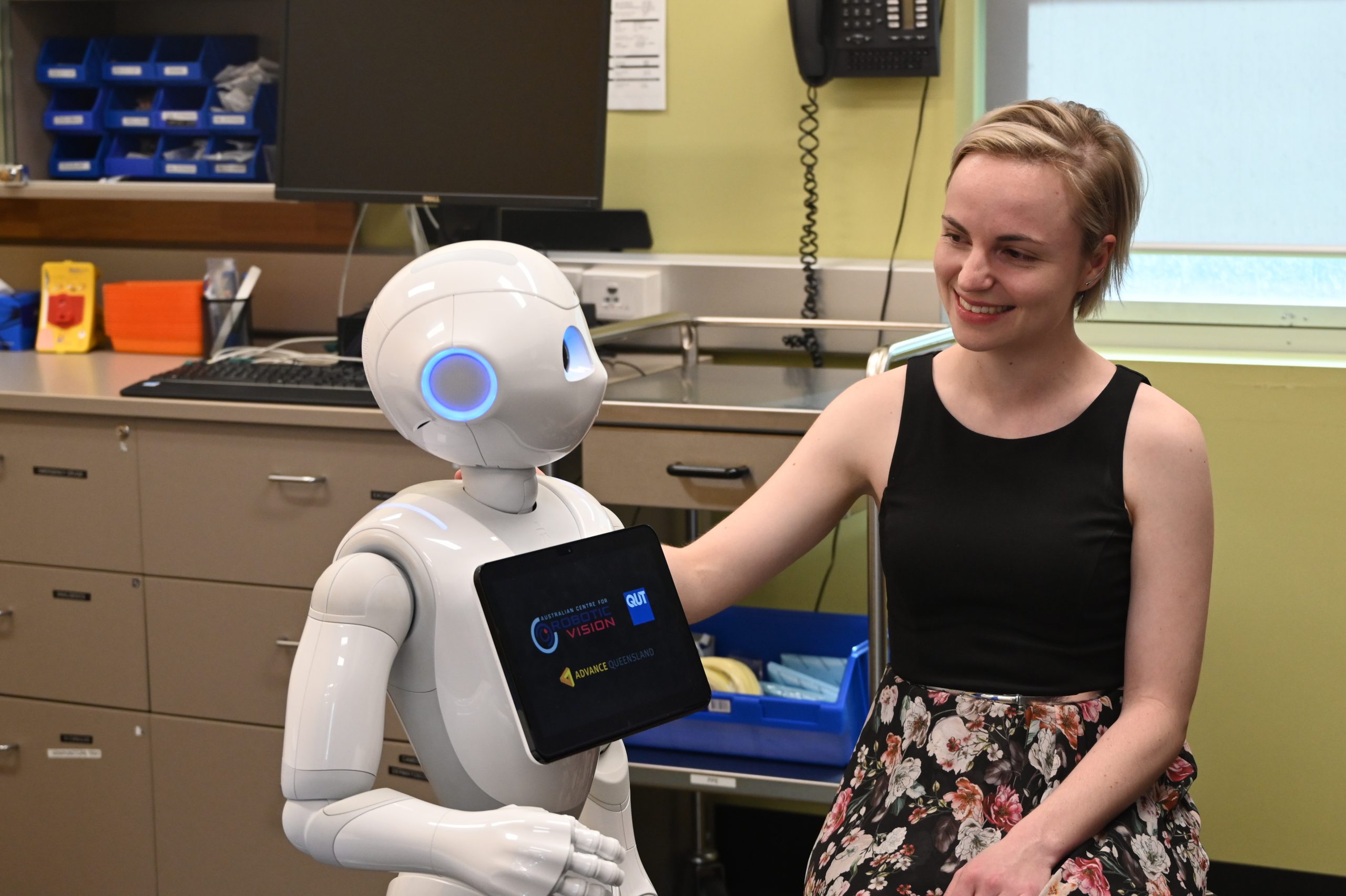
Prescribing a Social Robot ‘Intervention’
As our world struggles with mental health and substance use disorders affecting 970 million people and counting (according to 2017 figures), the time is ripe for meaningful social robot ‘interventions’. That’s the call by Centre Research Fellow Nicole Robinson – a roboticist with expertise in psychology and health.
After leading Australia’s first study into the positive impact of social robot interventions on eating habits, in 2017, Dr Robinson believes it’s time to focus on weightier health and wellbeing issues, including depression, drug and alcohol abuse, and eating disorders.
In a 2019 paper, A Systematic Review of Randomised Controlled Trials on Psychosocial Health Interventions by Social Robots, published in the Journal of Medical Internet Research (JMIR), Dr Robinson reveals global trials are ‘very few and unsophisticated’.
Only 27 global trials met inclusion criteria for psychosocial health interventions; many of them lacked a follow-up period; targeted small sample groups (<100 participants); and limited research to contexts of child health, autism spectrum disorder (ASD) and older adults.
Of concern, no randomised controlled trials involved adolescents or young adults at a time when the World Health Organisation (WHO) estimates one in six adolescents (aged 10-19) are affected by mental health disorders. According to the agency, half of all mental health conditions start by 14 years of age but most cases are undetected and untreated.
Despite limited global research conducted on psychosocial health interventions by social robots, Dr Robinson believes the results are nevertheless encouraging. They indicate a ‘therapeutic alliance’ between robots and humans could lead to positive effects similar to the use of digital interventions for managing anxiety, depression and alcohol use.
“Our research is not about replacing healthcare professionals, but identifying treatment gaps where social robots can effectively assist by engaging patients to discuss sensitive topics and identify problems that may require the attention of a health practitioner,” Dr Robinson said.
In 2019, she led a three-month research trial involving SoftBank’s Pepper robot at a QUT Health Clinic. The trial measured the unique value of social robots in one-to-one interactions in healthcare. It followed the Centre’s support of an Australia-first trial of a Pepper robot at Townsville Hospital and Health Service in 2018.
In the latest trial, Pepper delivered a brief health assessment and provided customised feedback to members of the public registered as patients at the QUT Health Clinic. The feedback was provided in confidence, giving patients a print-out and the option to discuss any concerns around physical activity, dietary intake, alcohol use and smoking with their health practitioner.
All of this research is part of the Humanoid Robotics Research and Development Partnership Program funded by the Queensland Government, who have provided $.1.5 million in funding over three years from 2017 to 2020, in an effort to explore and develop new and disruptive technologies and solutions in social and humanoid robotics.
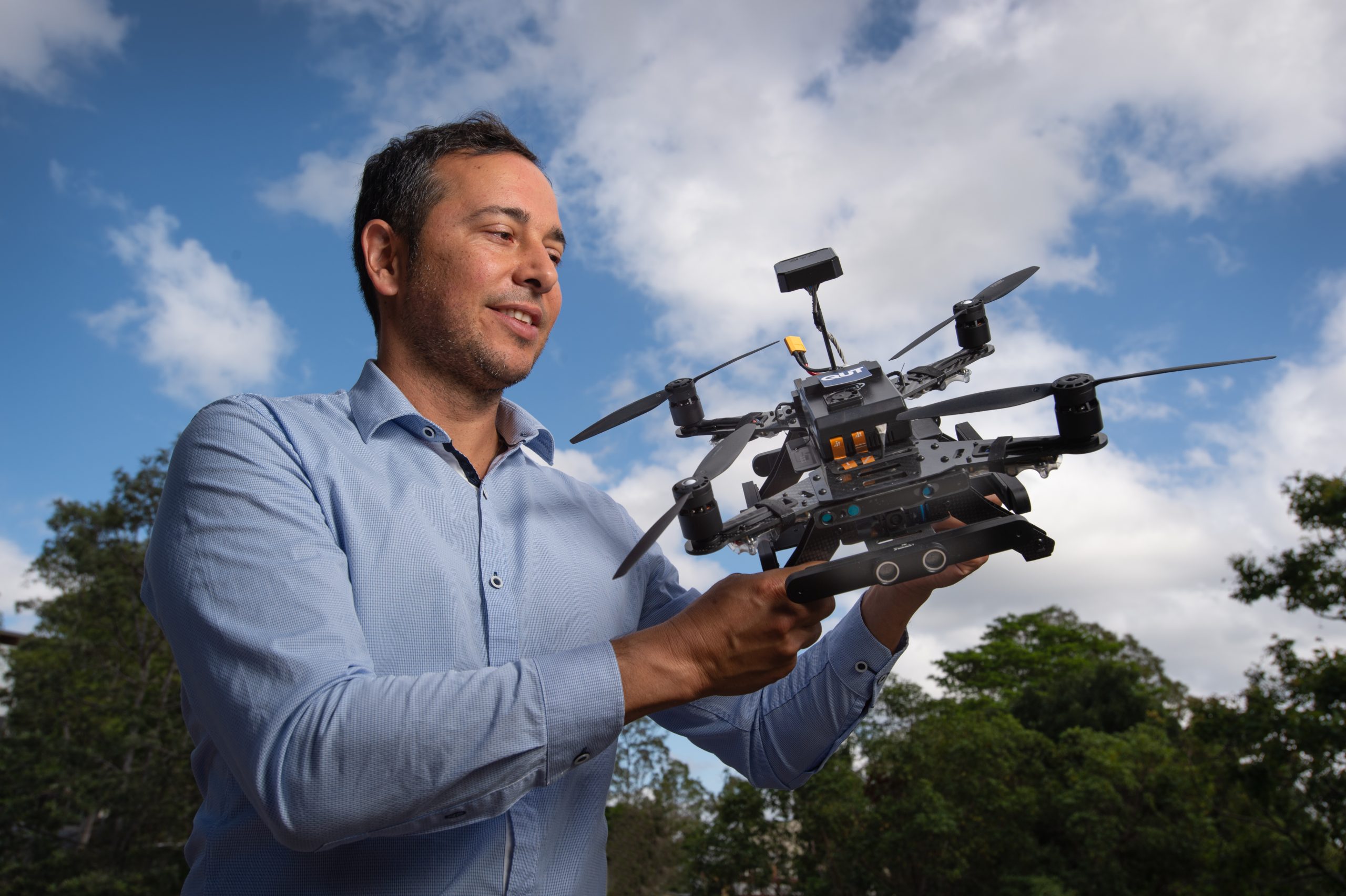
AI Reaches New Heights
Tech giant Microsoft has thrown its weight behind a research project led by Centre Associate Investigator Felipe Gonzalez that marries artificial intelligence, flying robots and conservation of the Great Barrier Reef.
In April 2019, Associate Professor Gonzalez, an aeronautical engineer based at QUT, became one of the grant recipients of Microsoft’s US$50 million AI for Earth program. The program recognises projects that ‘use AI to address critical areas that are vital for building a sustainable future’.
The grant enables Associate Professor Gonzalez to quickly process data using cloud computing services, saving weeks or perhaps months in data crunching time.
In partnership with the Australian Institute for Marine Science (AIMS), he has captured data from drones flying 60m above the Great Barrier Reef at four vulnerable reef locations.
The Great Barrier Reef stretches 2,300km with about 3,000 reefs, making the challenge of monitoring the condition enormous.
Associate Professor Gonzalez said: “One of the greatest challenges in saving the reef is its size – it’s far too large for humans to monitor comprehensively for coral beaching, and satellites and manned aircraft can’t achieve the ultra-high resolution that we can with our low-flying system.”
His drone system uses specialised hyperspectral cameras which, when validated using AIMS underwater data, can not only identify coral against the background of sand and algae, but also determine the type of coral and precise levels of coral bleaching.
A standard camera detects images in three bands of the visible spectrum of red, green and blue. The hyperspectral camera, flying over the reef, uses 270 bands of the visible and near-infrared spectrum – providing far more detail than the human eye can see.
Associate Professor Gonzalez launched the first flight project in late 2016, using the drones to analyse the health of four coral reefs in the Great Barrier Reef Marine Park.
Eighteen months later, when the Microsoft AI for Earth grant was announced, he had only processed about 30 per cent of the data the drones collected.
By the end of 2019, the grant allowed him to process 95 per cent of the data using Microsoft’s Azure cloud computing resources, including AI tools. The remaining five per cent will completed in 2020.
“You can’t just watch hyperspectral footage in the same way we can watch a video from a standard camera – we must process all the data to extract meaning from it,” he said.
“We’ve built an artificial intelligence system that processes the data by identifying and categorising the different ‘hyperspectral fingerprints’ for objects within the footage. Every object gives off a unique hyperspectral signature, like a fingerprint.
“The signature for sand is different to the signature for coral and, likewise, brain coral is different to soft coral. More importantly, an individual coral colony will give off different hyperspectral signatures as its bleaching level changes, so we can potentially track those changes in individual corals over time.”
Did you know? In 2019, Associate Professor Gonzalez’s drone system, equipped with machine learning and hyperspectral cameras, was displayed to industry stakeholders and the general public at the Royal Queensland Show’s inaugural Innovation Hub (9-18 August 2019). Outside work on the reef, the flying robots could become a new tool for the grape industry, protecting vineyards from what has been described as ‘the world’s worst grapevine pest’. Associate Professor Gonzalez has already demonstrated success using the drones, fitted with advance sensing equipment including multispectral, hyper-spectral, LiDAR and gas sensors, to detect grape phylloxera (Daktulospaira vitifoliae) in a research trial between QUT, Agriculture Victoria and the Plant Biosecurity CRC (PBCRC). Phylloxera is an aphid-like insect. Find out more
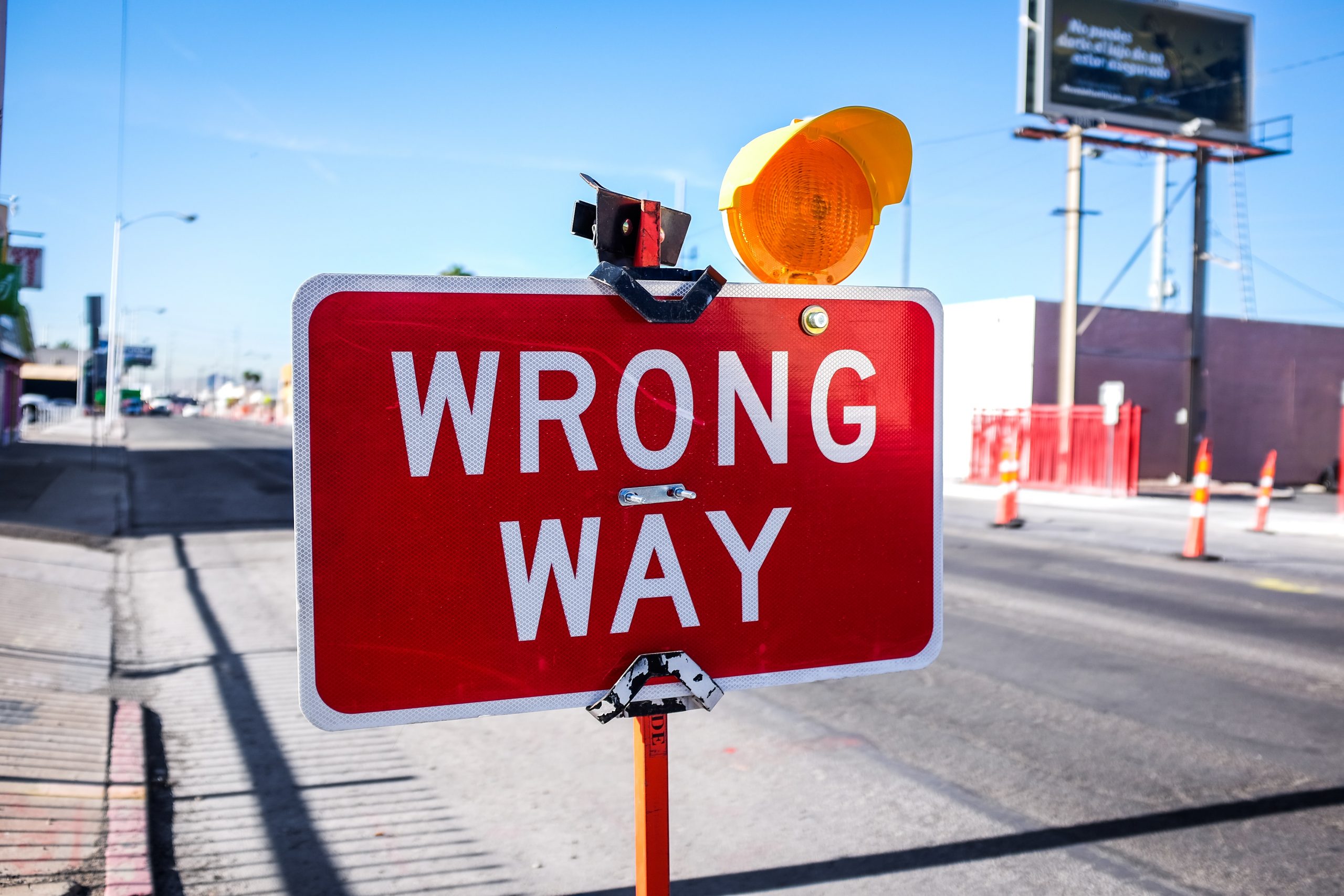
How Do Robots ‘Find’ Themselves?
Centre Research Fellow Yasir Latif, who jointly leads the Scene Understanding project from our University of Adelaide node, is convinced robots and humans share an innate ability to ‘find themselves’ based on what they’ve already seen. Dr Latif’s research focuses on the problem of place recognition and how robotic vision holds the key to the future success of autonomous vehicles when it comes to accurate localisation. In this case study, he explains the wider challenge of scalable place recognition.
Holidays usually last a week or two and involve taking continuous holiday snaps. Finding one particular image on your phone, however, can prove tricky, and may require a mental playback of the sequence of events that led to taking the photo. This is somewhat similar to mentally navigating to a location you’ve been to once or twice before, but without the help of GPS.
For humans, this can be frustrating, but not impossible. We intuitively replay a sequence of mental images that provide information about our location at any one point in time. This is called place recognition.
Now, imagine if your mobile phone never stopped capturing images, day and night. It would be virtually impossible to navigate through all the content to find one image from a sequence of holiday snaps taken weeks, months or maybe years ago.
This is precisely the problem autonomous vehicles have to solve. In place of photos on a mobile phone, these robots must make sense of a continuous stream of video sequences (equivalent to millions of images) captured while in motion.
As a result, for robots, successful localisation over large scale observations happens through a ‘scalable’ version of place recognition that we call scalable place recognition.
Autonomous cars will soon become a reality on our roads. To navigate safely and make sound decisions ‘on the go’, self-driving cars will need to make sense of a never-ending sequence of images as quickly as possible.
As part of the Centre’s Scene Understanding research project, we’re applying the same ‘human’ strategy of place recognition to robots, except on a larger scale. We use scalable place recognition to match what a robot is actively seeing ‘on the go’ to millions of previously observed images.
Individual images may not be informative enough for localisation. However, gathering bits and pieces of evidence from each image and using that to reason over sequences has shown great promise for localisation, even when the appearance of images changes due to weather, time of day, etc.
Ultimately, we want to extend the reasoning of place recognition to continuous observation for the life-time of a robot, as will be required for autonomous vehicles.
A more immediate problem, however, is storage. All the images seen by an autonomous vehicle need to be stored in memory to enable sequential reasoning about them.
We all know how quickly our mobile phones fill up with images and videos. Our research is tackling this problem from a memory scalability perspective. We want to combine what we know presently about our location to make predictions about where we will be in the next instant in time. Using this information, we can then limit the set of images that we need to reason over.
We are hopeful that by combining sequential analysis with memory management, we will be able to achieve a method that enables an autonomous vehicle to localise itself over arbitrarily large image collections.
One thing’s for sure. It’s an exciting time to be involved in robotics research, particularly in the new field of robotic vision.
Imagine this future scenario: autonomous cars that can work together to capture a real-time snapshot of what the world looks like at any given moment and how it changes from day to day. Such large-scale place recognition methods could enable precise localisation for each self-driving car by looking at the world through the eyes of all cars. Exciting times, indeed!
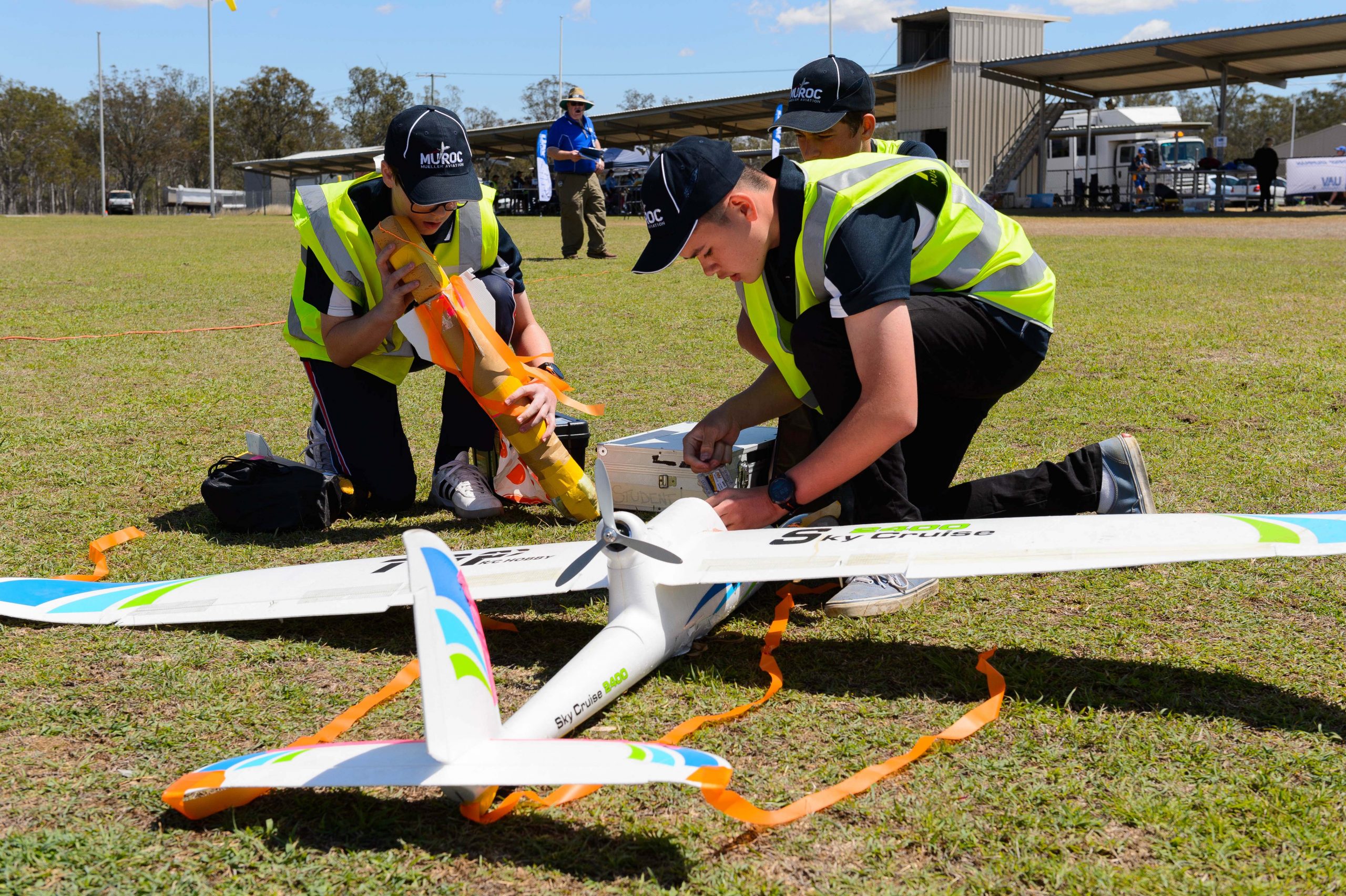
Flying Robots to the Rescue
The world’s biggest outdoor airborne robotics challenge, the UAV Challenge, staged its 13th annual high school competition in the rural Queensland town of Calvert (12-13 October, 2019).
Co-organised by CSIRO’s Data61 and the Australian Centre for Robotic Vision’s QUT node, the initiative also includes an open competition, held every two years, and next scheduled for 2020.
The UAV Challenge stakes its claim as ‘the world’s biggest outdoor UAV competition’ based on the number of global teams involved.
In 2019, a group of Californian students were crowned champions of the annual high school-level competition, the Queensland Government Airborne Delivery Challenge, from a field of 14 teams coming from across Australia and the United States. This marked the second year in a row a team representing William J. ‘Pete’ Knight High School in Palmdale, California, won the competition.
Queensland teams from Mueller College in Rothwell and Marist College in Ashgrove were placed second and third respectively, with Marist College students also claiming a new ‘Innovation Award’ for use of robotic vision onboard their UAV.
Since its launch in 2007, the UAV Challenge has drawn an eye-boggling array of agile, low-cost UAV lifesavers. Flying robots of all shapes and sizes, weighing-in anywhere from 3kg (the weight of a brick) to around 20kg (think three bowling balls or checked-in luggage) and resembling everything from mini planes to UFO-like contraptions.
The high school competition tasks students with delivering a life-saving medical device (an EpiPen) to ‘Outback Joe’, a mannequin simulating a real person in need of urgent medical attention.
The open, biennial Medical Rescue competition takes on a new format in 2020. Find out more
Centre Chief Investigator Jonathan Roberts is co-founder of the UAV Challenge. He said: “The UAV Challenge has inspired advances in drone design as well as software and communications systems, not least being enhancements to the functionality and codebase of open source autopilot software, ArduPilot, which is now embraced by major players such as Microsoft and Boeing.
“We hope it will continue to push the UAV community further and continue our own mission of accelerating the use of unmanned aircraft and systems that can save lives.”
The UAV Challenge is supported and sponsored by the Queensland Government, Insitu Pacific and Boeing, Northrop Grumman, the Australian Government’s Department of Defence, CASA, the Australian Association for Unmanned Systems, Nova Systems and QinetiQ.
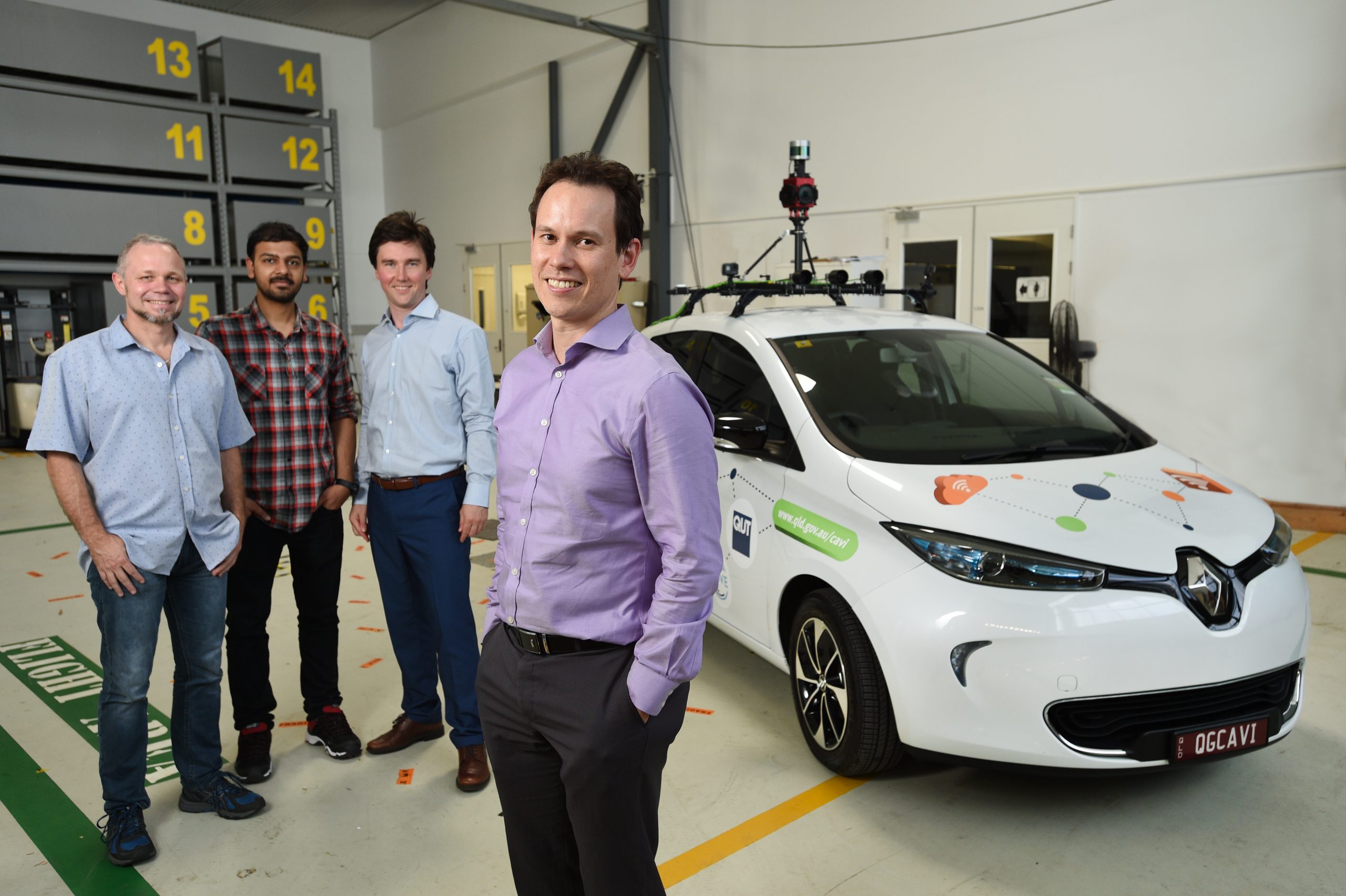
Connected and Highly Automated Driving (CHAD) Pilot
Artificial intelligence (AI) took to the road in 2019 as the ultimate back-seat driver.
Centre Chief Investigator Michael Milford led a team of researchers in a novel, tech-savvy project aimed at ensuring autonomous cars of the future will be smart enough to handle tough Australian road conditions.
The project, ‘How Automated Vehicles Will Interact with Road Infrastructure Now and in the Future’, is part of the CHAD Pilot funded by Queensland Department of Transport and Main Roads (TMR), QUT, Motor Accident Insurance Commission (MAIC); and iMOVE Cooperative Research Centre (an Australian Government initiative).
It was launched by Queensland Minister for Transport and Main Roads Mark Bailey in February 2019.
The project involved a driver taking an electric Renault research vehicle, dubbed ZOE1, on a 1,286km road trip, traversing a wide range of road and driving conditions.
The ZOE1 has all the sensors and computers of an autonomous vehicle including LIDAR, cameras, precision GPS and other onboard systems.
“We took ZOE1 on a road trip around South-East Queensland to assess the ability of current state-of-the-art open source technologies for autonomous vehicle-related competencies like sign detection and recognition, traffic light detection, lane marker detection and positioning,” said Professor Milford.
“We covered metropolitan, suburban and rural roads, under a wide range of conditions including day, night and rain. We encountered lots of interesting sections of road, including sections that have been completely dug up for construction and were no more than dirt roads.
“This was a fantastic team effort, involving a number of staff from TMR, the iMOVE CRC and from QUT, including centre-associated researchers James Mount and Sourav Garg, as well as research assistants Nikola Poli and Matthew Bradford. We’re discussing a range of potential future projects in this exciting and highly relevant topic.”
Full findings of the project, which wrapped up at the end of 2019, will be released in 2020.
Professor Milford outlines initial findings below:
Poor performance of ‘state-of-the-art’ technology ‘out of the box’: “It didn’t work very well, despite being the best available in the research community. It had to be trained and tuned to Australian roads and conditions.”
Previously established high-quality maps of the environment are critical: “The car can use these prior maps to achieve significant improvements in the performance of its onboard systems. In essence, it knows what to expect, and is able to look for and confirm (or sometimes disprove) those observations. Maps will likely go a long way towards solving another problem we found which was context. It’s very hard to determine which signs are relevant for the car. For example, traffic control lights on the other side of the road or on parallel train tracks can be very confusing.
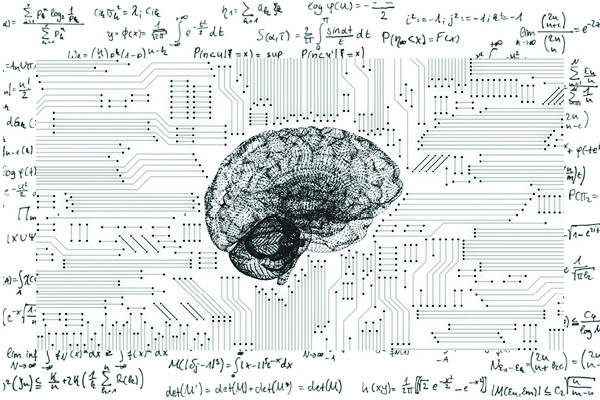
Embracing Chaos: VSLAM 101 for Real-World Robots on the Move!
The Centre’s ultimate goal is for robotic vision to become the sensing modality of choice, opening the door to affordable, real-world robots.
Centre PhD Researcher Pieter van Goor is developing a ground-breaking algorithm that takes a real-world (non-linear) approach to help robots see and understand just like humans. The genius, according to Pieter, comes in understanding that few things are straight-forward or precisely predictable in complex and dynamically-changing environments of the real world.
As a result, robots – in particular mobile robots like autonomous cars or unmanned aerial vehicles (UAVs) – need systems that work off algorithms more closely matched to chaos theory than constant, predictable linear assumptions of a ‘perfect world’.
Under the Centre’s Fast Visual Motion Control project, Pieter is developing a Visual Simultaneous Localisation and Mapping (VSLAM) system of an entirely new class.
VSLAM systems enable a robot or device equipped with a single standard camera to estimate its motion through its environment, and to understand the structure of its surroundings.
“Our VSLAM system is an entirely new class because we treat the non-linear problem as a non-linear problem, and we don’t assume that uncertainty is described with normal distributions,” Pieter said.
“This means that these assumptions don’t get in the way of results. In other systems, when the assumptions are violated, they just stop working. Ours won’t do that.”
By way of example, a common problem in traditional VSLAM systems occurs when a mobile robot equipped with a camera rotates too quickly. This is because rotation is a very non-linear action, but the traditional VSLAM algorithms try to treat it as a linear one.
Quite a few of these systems stop working or break if the robot’s camera rotates too fast. By contrast, the system developed by Pieter treats non-linear actions exactly as they are: non-linear.
“This means that even after a large rotation, our algorithm won’t fail or break,” he said.
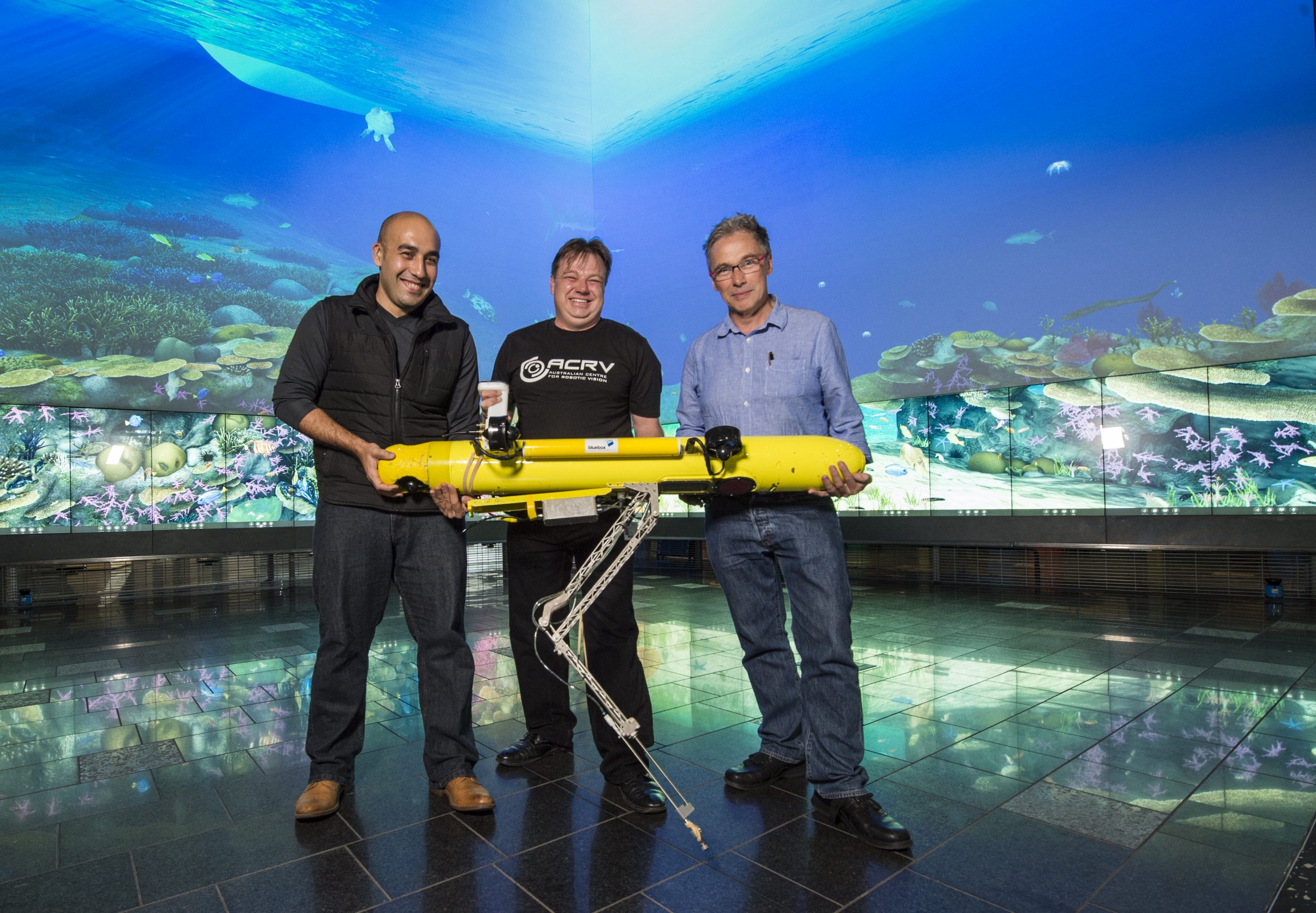
Could this be the World’s Most Decorated Marine Robot?
The world’s first robotic-vision empowered coral reef protector, RangerBot, designed specifically to help safeguard coral reef environments, collected a trifecta of accolades in the 2019 Good Design Australia Awards.
RangerBot won the Good Design Award for Sustainability; the Museum of Applied Art and Sciences Award; and Good Design Award Best in Class Accolade (Product Design category).
Australia’s annual Good Design Awards program is one of the oldest and most prestigious international design awards in the world, promoting excellence in design and innovation since 1958. It is recognised by the World Design Organization (WDO) as Australia’s peak international design endorsement program.
The story behind RangerBot
Centre Chief Investigator Matthew Dunbabin is the mastermind behind RangerBot, which claimed the 2016 Google Impact Challenge People’s Choice prize as a high-tech, low-cost autonomous underwater robot.
The multipurpose ‘drone of the ocean’ evolved from Professor Dunbabin’s larger, single-purpose COTSbot prototype that was programmed to visually recognise and record populations of crown-of-thorns starfish (COTS).
First trialled in 2015, COTSbot’s design included an automated system to inject detected COTS with a solution to control their numbers.
“To find crown-of-thorns starfish in a reef environment is incredibly difficult,” said Professor Dunbabin.
“If a robot is looking for a chair, for instance, that chair will probably have a lot of context around it – in a room with a floor, flat walls and things. But if you put the chair in a forest, no part of that forest will ever look the same as any other.
“That’s the problem we have on coral reefs. We had to reduce that problem down to something that’s manageable.
“We generated models of the starfish by training the robot on hundreds of thousands of images collected from many reefs under different lighting and visibility conditions. These models allow the robot to quickly and robustly detect the starfish in new, previously unvisited locations on the reef.”
RangerBot is a more versatile, cost-effective version of COTSbot.
The 15kg robot is the result of Professor Dunbabin and fellow Centre Chief Investigator Feras Dayoub teaming up with the Great Barrier Reef Foundation, in 2016, to enter the Google Impact Challenge. They won the People’s Choice accolade and secured $750,000 to develop RangerBot.
First trialled on the Great Barrier Reef in 2018, RangerBot surpassed the capabilities of COTSbot, taking the initial concept to an entirely new dimension with autonomous rather than tethered operation; a high tech vision system; enhanced mobility (including multiple thrusters allowing movement in every direction); and the ability to monitor reef health.
“RangerBot is the world’s first underwater robotic system designed specifically for coral reef environments, using only robotic vision for real-time navigation, obstacle avoidance and complex science missions,” Professor Dunbabin said.
“This multifunction ocean drone can monitor a wide range of issues facing coral reefs including coral bleaching, water quality, pest species, pollution and siltation. It can help to map expansive underwater areas at scales not previously possible, making it a valuable tool for reef research and management.
“RangerBot can also stay underwater almost three times longer than a human diver, gather more data, and operate in all conditions and at all times of the day or night, including where it may not be safe for a human diver.”
Romancing ‘the Reef’
In 2018, RangerBot was put to work, in a world-first for robots, delivering coral larvae in a coral seeding program on the Great Barrier Reef timed during the annual splendour of coral spawning.
The ongoing coral seeding project – involving collaboration with QUT and Professor Dunbabin – is led by Southern Cross University marine biologist Peter Harrison. The partnership, which could revolutionise coral restoration on reefs worldwide, is funded by the Great Barrier Reef Foundation with support from The Tiffany & Co Foundation.
In 2019, Professor Dunbabin scaled up his robotic fleet, returning to the Great Barrier Reef with two RangerBot-turned-LarvalBots and an inflatable LarvalBoat.
“In the year since the first LarvalBot trial, we’ve been able to extend the reach of the robot’s larval delivery system from 500 square metres to a recent trial in the Philippines where a LarvalBot was able to cover an area of three hectares of degraded reef in six hours,” Professor Dunbabin said.
“We are expanding the LarvalBot fleet which can be fitted with a range of payloads. We’ve had significant interest from around the world to use LarvalBots to spread coral larvae where it’s most needed, and we’ve designed the new LarvalBoat inflatable system so in future they can be fitted in backpacks and the two can be transported and used together.”
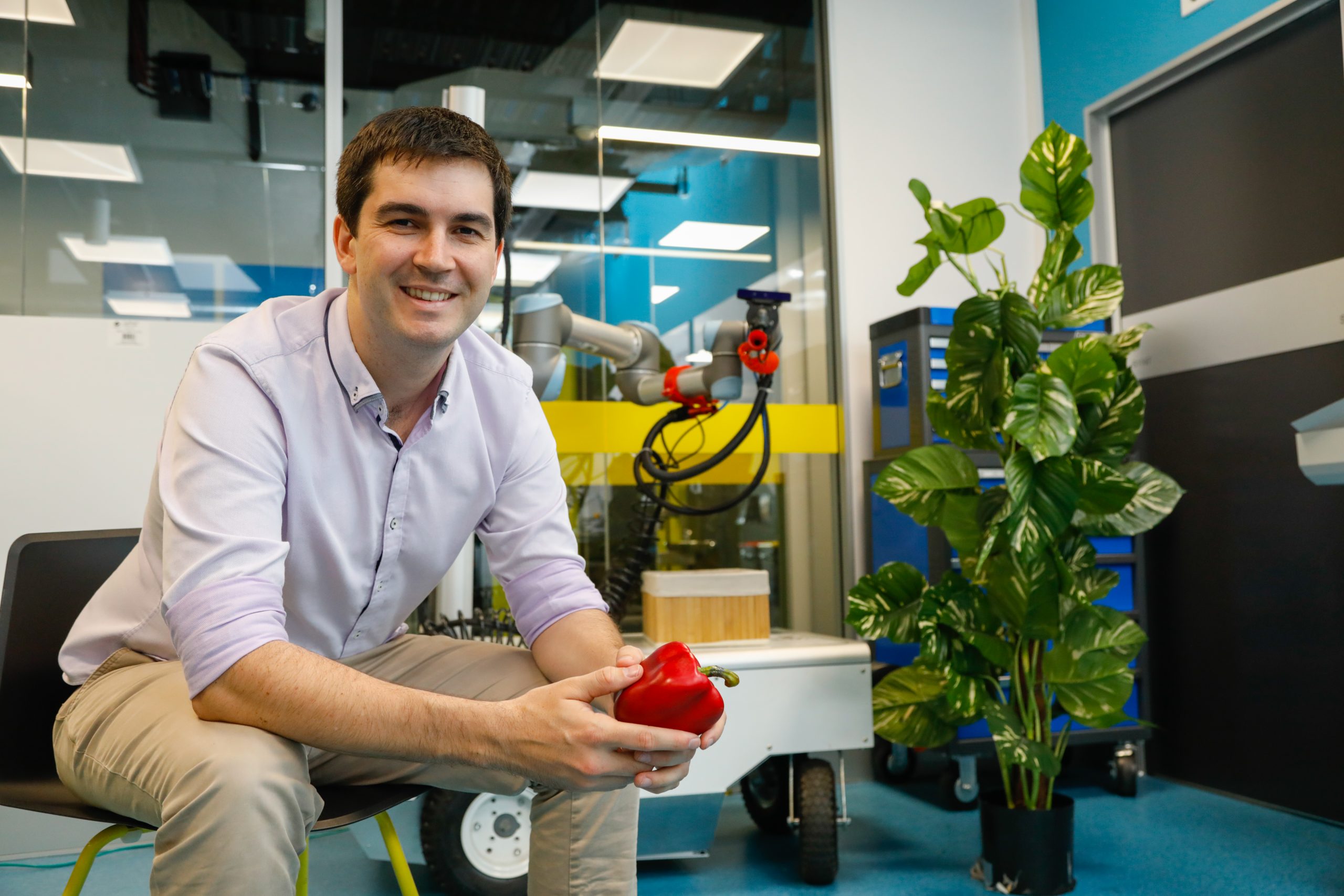
Farm-Hand Robots
With the global population projected to reach 9.8 billion in 2050, the Australian Centre for Robotic Vision is focused on giving the next generation of robots the vision and understanding to help solve real-world challenges, including sustainable food production.
In March 2019, Centre Associate Investigator Chris Lehnert stepped up as part of QUT’s involvement in a new Future of Food Systems Cooperative Research Centre (CRC).
Dr Lehnert is recognised for developing one of the world’s best robotic harvesters called Harvey. His role at the CRC will focus on further development of robotics and smart technology for vertical and indoor protected cropping.
The Future of Food Systems CRC is backed by $35 million in Federal Government funding over 10 years, plus $149.6 million in cash and in-kind funds from more than 50 participants.
As detailed in Australia’s first Robotics Roadmap, released by the Australian Centre for Robotic Vision in 2018, agriculture is Australia’s most productive sector, outstripping the rest of the economy by a factor of two.
AgTech is positioned to become Australia’s next $100bn industry by 2030. Key robotic technologies for the sector include autonomous vehicles, connected devices, sensor networks, drones and robotics.
As in all industries, robotic vision expands the capabilities of robots to work alongside people and adapt to complex, unstructured and dynamically changing environments of the real world.
According to Dr Lehnert, autonomous, vision-empowered robots, like Harvey, have the potential to assist fruit and vegetable farmers across Australia who often experience a shortage in skilled labour, especially during optimal harvesting periods.
“The future potential of robotics in indoor protected cropping will be their ability to intelligently sense, think and act in order to reduce production costs and maximise output value in terms of crop yield and quality,” Dr Lehnert said.
“Robotics taking action, such as autonomous harvesting within indoor protected cropping will be a game changer for growers who are struggling to reduce their production costs.”
The Future of Food Systems CRC was initiated by NSW Famers Association on behalf of the national representative farm sector and as part of a broader industry-wide push to increase value-adding capability, product differentiation and responsiveness to consumer preferences.
Are 9 eyes better than 1?
Not when one eye has the power of nine. That’s the upshot of a ground-breaking research project led by Dr Lehnert in collaboration with leading Defence industry avionics engineer Paul Zapotezny-Anderson.
In October 2019, the pair transformed Harvey into a robo-Cyclops of sorts in a project aimed at ensuring an autonomous robotic harvester won’t ‘roll off the job’ when leaves get in the way of fruit and vegetable picking.
“In robotic harvesting, dealing with obstructing leaves and branches that stop a robot from getting a clear view of a crop is really tricky because the ‘occlusions’ are extremely difficult to model, or anticipate, in a technical way,” said Mr Zapotezny-Anderson.
“Basically, robots don’t cope well and often just give up!”
This is no longer the case for Harvey thanks to the research collaboration with Mr Zapotezny-Anderson during his sabbatical from Airbus to complete a Masters of Engineering (Electrical Engineering) at QUT.
The project advanced Dr Lehnert’s initial 3D-printed visual servoing system equipped with nine ‘eyes’ (cameras) operating at different depths to enable Harvey to look around obstructing leaves much like a human.
The new, faster monocular-vision system, aptly entitled Deep 3D Move To See, retains the scope of multiple eyes.
Dr Lehnert said: “The real beauty of the one-camera system is that one eye has the power of nine, without the performance limitations of a multi-camera system… not least being slower data processing time.”
Mr Zapotezny-Anderson added: “Basically, Harvey has been trained off all nine cameras to use monocular vision to guide its end effector (harvesting tool or gripper) on the fly around occluding leaves or branches to get an unblocked view of the crop, without prior knowledge of the environment it needs to navigate in. Perhaps a good way of thinking about it is that Harvey can now harvest crops with one eye open and eight eyes closed!”
Deep 3D Move To See is the result of a novel ‘deep learning’ method involving one camera being trained off nine via a Deep Convolutional Neural Network. A related paper, Towards active robotic vision in agriculture: A deep learning approach to visual servoing in occluded and unstructured protected cropping environments, was presented at the 6th IFAC Conference on Sensing, Control and Automation Technologies for Agriculture.
Watch Harvey in action
*NOTE: While the 3D-printed camera housing attached to Harvey’s gripper retains nine cameras (as seen in the video taken during rigorous trials at the Centre’s QUT-based Lab), Harvey now relies on only one camera, positioned in the centre of the array.
Did you know? In 2019, Harvey was demonstrated to industry stakeholders and the public at the Royal Queensland Show’s inaugural Innovation Hub (9-18 August 2019), and at QODE (2-3 April 2019), an innovation and technology event based in Brisbane targeting entrepreneurial start-up and investment communities.
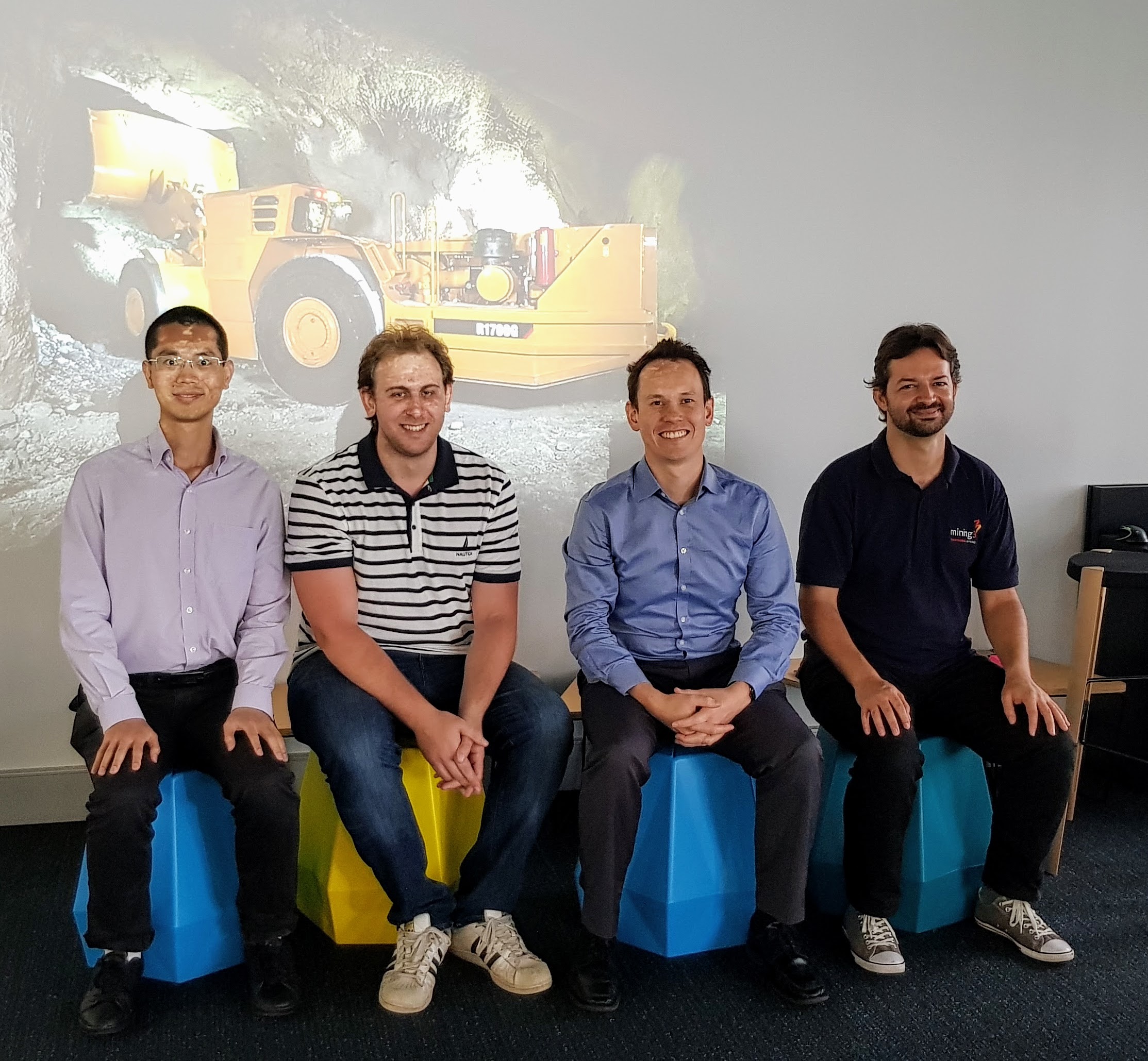
Robotic Vision and Mining Automation
In collaboration with Caterpillar, Mining3 and the Queensland Government, QUT-based Centre researchers have successfully developed and tested new technology to equip underground mining vehicles to navigate autonomously through dust, camera blur and bad lighting.
The team led by Centre Chief Investigator Michael Milford has undertaken a total of six field trips to Australian mine sites. Their work involved development of a low-cost approximate positioning system for tracking mining vehicle inventory underground.
In the final stage of the project (September 2019), the team undertook a major field trip to an underground mine where the positioning system was tested live in a challenging multi-day trial.
“The system performed well and the data and insights from the final trial are assisting in Caterpillar’s ongoing commercialization and refinement process,” said Professor Milford.
The team presented a final conference paper, TIMTAM: Tunnel-Image Textually-Accorded Mosaic for Location Refinement of Underground Vehicles with a Single Camera at the IEEE/RSJ International Conference on Intelligent Robots.
Two key research staff from the project, Adam Jacobson and Fan Zeng have moved into Brisbane-based roles with industry partner Caterpillar and sensor technology company, Black Moth, which provided some of the equipment used in the project.
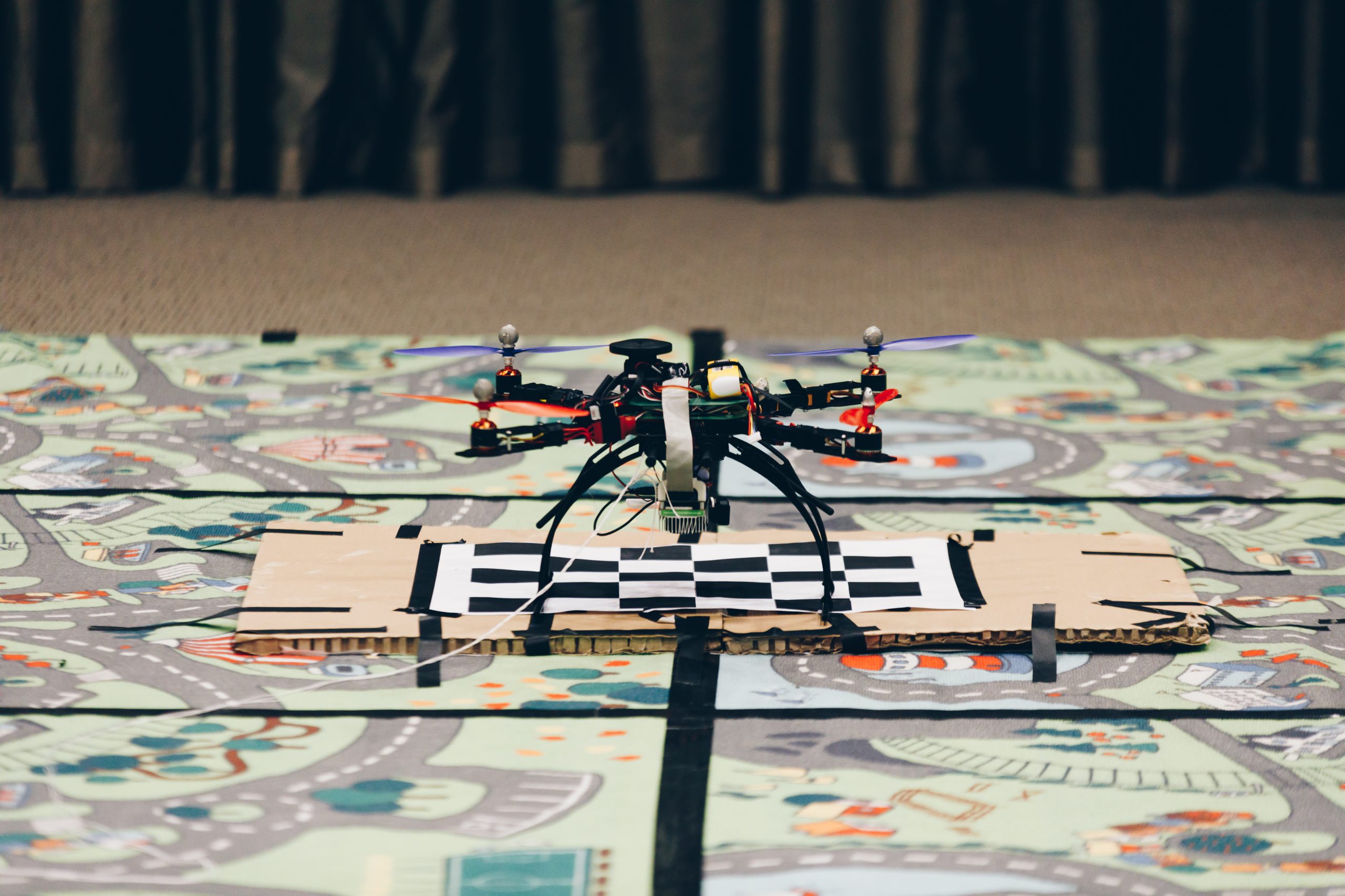
What do Flying Robots and Star Wars Speeder Bikes have in Common?
Star Wars speeder bikes, able to manoeuvre through forests at break-neck speed without crashing into trees, are on the wish-list of Centre researchers.
According to Research Fellow Yonhon Ng, there’s good reason to develop vision algorithms and control technology to achieve similar feats with small-scale aerial robots.
“The relationship between motion in the world and changes in images are at the heart of robotic vision, providing rapid and continuous feedback for control,” said Yonhon.
“We’re a long way from autonomous flying robots successfully performing like Star Wars speeder bikes, but anything’s possible in the future!”
In the interim, Yonhon is focused on high-performance control of a small (sub 800g) quadcopter. The goal is for the quadcopter to autonomously perform challenging acrobatic manoeuvres like 360-degree flips or flying through a window.
The appeal of the quadcopter as an agile robotic platform is its suitability for a wide range of real-world applications. For example, a quadcopter can be used to enter a collapsed building to search for survivors after a natural disaster.
Due to the agility of the quadcopter, the research project uses vision (including event cameras) and inertial sensors to allow for high-speed autonomous navigation within an unstructured environment.
Yonhon and fellow researchers on the Fast Visual Motion Control project have built a quadcopter with frame and motors from the popular Hawk 5, pixracer flight controller and Turnigy Multistar BLHeli_32 ESC.
The ANU has an indoor flying space equipped with VICON positioning system for controlled lab experiments.
In collaboration with ArduPilot developer Dr Andrew Tridgell, the project group has updated the open-source autopilot software to integrate VICON position measurements into the onboard EKF-based state estimation for indoor flight.
In 2019, they succeeded in getting the modified Hawk 5 quadcopter to fly a 1m circle at 1/3 revolution a second (watch video).
“An interesting research problem we’ve encountered is the presence of sensor lag,” Yonhon said.
“For a fast-moving vehicle, the accurate determination of the sensor lag is vital to align different sensor measurements (e.g. IMU, GPS/VICON, vision) for correct state estimation. Traditionally, the sensor lag is assumed constant and calibrated for a specific sensor type.
“However, in practice, this sensor lag is time-varying. For example, the sensor lag for GPS measurement changes depending on the number of satellites, different constellations etc. To overcome this problem, we are developing a method to estimate the sensor lag in real-time to improve state estimation.”
The group’s next performance task for the quadcopter is to achieve fast, autonomous acrobatic manoeuvres (e.g. flips, flying through a small window) using VICON position and inertia measurements.
“Once we are satisfied with the quadcopter’s performance, tested indoors, the next step is to improve its capabilities to work in challenging outdoor environments, performing the same task using only inertial measurements and vision with the help of unconventional sensors like event cameras,” Yonhon said.
“Solving this problem will help progress the state-of-the-art algorithm in fast, six degrees-of-freedom pose estimation in GPS-denied (and VICON-denied) environments.”
Did you know? Event cameras operate similarly to human eyes and do not suffer from delay. Like the human eye, they capture visual information asynchronously and continuously without relying on a shutter to capture images. Importantly, event cameras are not susceptible to motion blur, which is a big problem for conventional cameras, especially in high-speed, low-light scenarios.