Scene Understanding
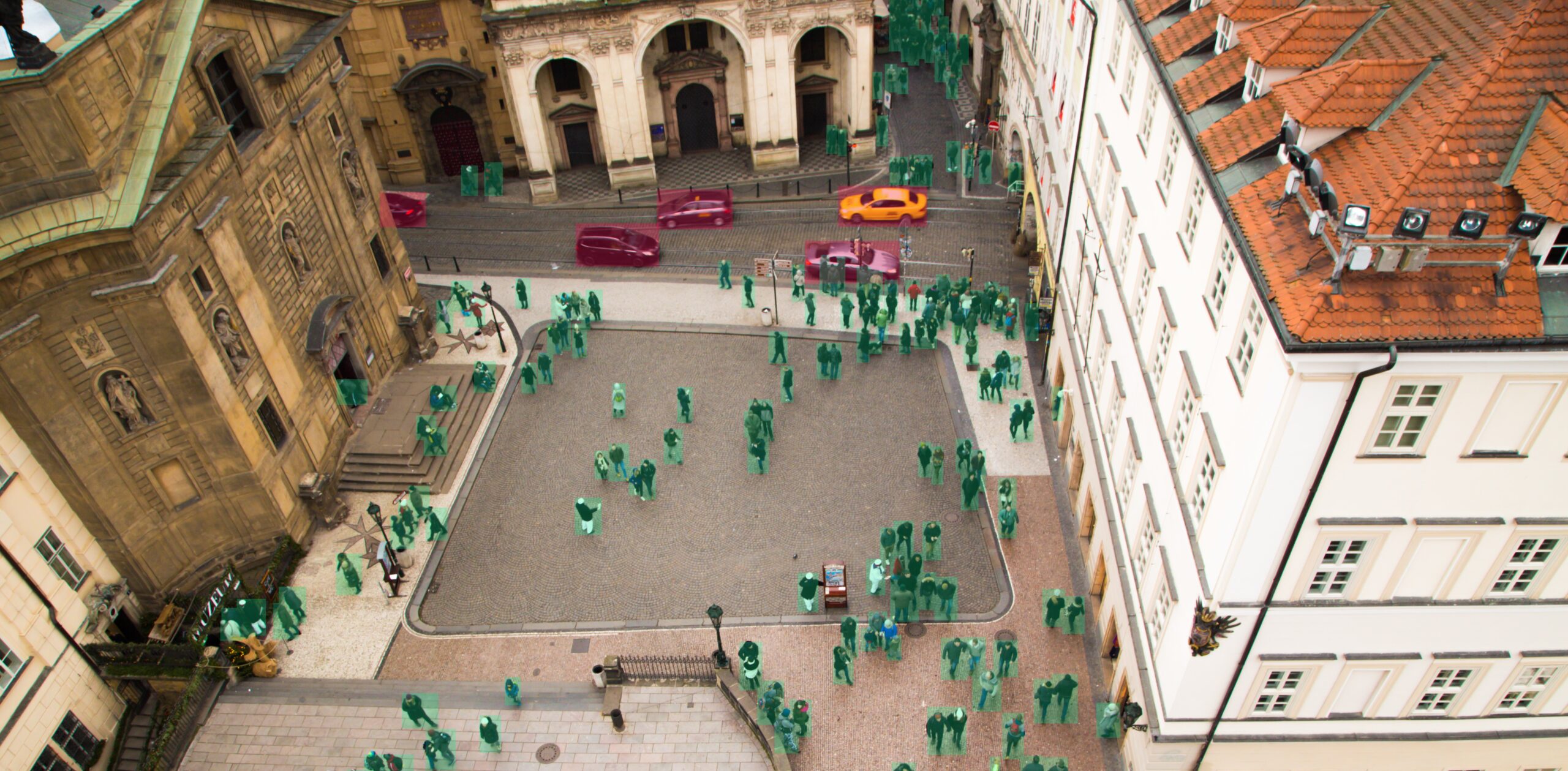
The purpose of the Scene Understanding project is to create geometric and semantic models and representations of an environment from visual observations. This ability is crucial for a robotic system to reason about a scene, its objects and their relationships, and their affordances, so that it can plan actions effectively.
Team Members
Niko Sünderhauf
Queensland University of Technology
Associate Professor Niko Suenderhauf is a Chief Investigator at the Centre where he leads the Robotic Vision Evaluation and Benchmarking project. As a member of the Executive Committee, Niko leads the Visual Learning and Understanding program at the QUT Centre for Robotics. Niko conducts research in robotic vision, at the intersection of robotics, computer vision, and machine learning. His research interests focus on scene understanding and how robots can learn to perform complex tasks that require navigation and interaction with objects, the environment, and humans.
Associate Professor Suenderhauf is co-chair of the IEEE Robotics and Automation Society Technical Committee on Robotic Perception and regularly organises workshops at leading robotics and computer vision conferences. He is member of the editorial board for the International Journal of Robotics Research (IJRR), and was Associate Editor for the IEEE Robotics and Automation Letters journal (RA-L) from 2015 to 2019. Niko served as AE for the IEEE International Conference on Robotics and Automation (ICRA) 2018 and 2020.
In his role as an educator at QUT, Niko enjoys teaching Introduction to Robotics (EGB339), Mechatronics Design 3 (EGH419), as well as Digital Signals and Image Processing (EGH444) to the undergraduate students in the Electrical Engineering degree.
Niko received his PhD from Chemnitz University of Technology, Germany in 2012. In his thesis, Niko focused on robust factor graph-based models for robotic localisation and mapping, as well as general probabilistic estimation problems, and developed the mathematical concepts of Switchable Constraints. After two years as a Research Fellow in Chemnitz, Niko joined QUT as a Research Fellow in March 2014, before being appointed to a tenured Lecturer position in 2017.
Tat-Jun Chin
University of Adelaide
Tat-Jun Chin received his PhD in Computer Systems Engineering from Monash University in 2007, which was supported by the Endeavour Australia-Asia Award, and a Bachelor in Mechatronics Engineering from Universiti Teknologi Malaysia in 2004, where he won the Vice Chancellor’s Award. He currently holds the SmartSat CRC Professorial Chair of Sentient Satellites at The University of Adelaide. He is also the Director of Machine Learning for Space at The Australian Institute for Machine Learning. Tat-Jun’s research interest lies in optimisation for computer vision and machine learning, and their application to robotic vision, space and smart cities. He has published more than 100 research articles on the subject, and has won several awards for his research, including a CVPR award (2015), a BMVC award (2018), Best of ECCV (2018), two DST Awards (2015, 2017) and IAPR Award (2019).
Yasir Latif
University of Adelaide
Yasir Latif did his bachelors at Ghulam Ishaq Khan Institute of Engineering Science and Technology in Topi, Pakistan and his master in Communication Engineering from Technical University of Munich (TUM), Germany. After that, he pursued his PhD at University of Zaraogoza, Spain under the supervision of Professor Jose Neira. He visited Imperial College London and Massachusetts Institute of Technology for short research stays during that period. The main theme of his doctoral thesis was reliable loop closure detection and verification for the Simultaneous Localization and Mapping (SLAM) problem.
His interests include SLAM, Computer Vision and looking for the ultimate question. Yasir has received various awards for his research including best student paper at ICRA 2015 and DICTA 2019.
Pulak Purkait
University of Adelaide
Pulak received a PhD in computer science from the Indian Statistical Institute (ISI), Kolkata, India, in 2014. He was a postdoctoral researcher with the University of Adelaide, from September 2013 to February 2016 and again from September 2018. He has spent two years (2016-2018) at Toshiba Research Europe, Cambridge UK before joining back at the University of Adelaide. His research interests include image processing, computer vision and machine learning. He joined the Centre in 2018 and is currently leading a project on 3D scene graph generation.
Ravi Garg
University of Adelaide
Ravi Garg is an Associated Research Fellow in the Centre and has been a senior research associate at the Australian Centre for Visual Technologies at The University of Adelaide since April 2014. He is working with Professor Ian Reid on his Laureate Fellowship project named “Lifelong Computer Vision Systems”. Prior to joining University of Adelaide, he finished his PhD at the Queen Mary University of London under the supervision of Professor Lourdes Agapito where he worked on Dense Motion Capture of Deformable Surfaces from Monocular Video.
His current research interest lies in building learnable systems with little or no supervision which can reason about scene geometry as well as semantics. He is exploring how far the visual geometry concepts can help current deep neural network frameworks in scene understanding.
Wei Liu
University of Adelaide
Wei Liu is a postdoctoral research fellow at The University of Adelaide working with Chief Investigator Ian Reid. Wei received his B.E degree from Xi’an Jiaotong University and Ph.D. degree from Shanghai Jiao Tong University in 2012 and 2018, respectively. His research interests mainly focus on image filtering in low-level computer vision and deep learning-based monocular SLAM systems.
Kejie ‘Nic’ Li
University of Adelaide
Kejie graduated from ANU with a Bachelor of Advanced Computing (Honours) with first class honours in 2016. During this time, he mainly worked on single view depth estimation. He joined the Centre in 2017 to work on semantic scene understanding, and the intriguing yet challenging task of building robots that are able to better interact with the world by well understanding the concept of objects and environment.
Huangying Zhan
University of Adelaide
Huangying is currently a PhD Student at the University of Adelaide and affiliated with the Australian Centre for Robotic Vision. He is advised by Professor Ian Reid and Professor Gustavo Carneiro. His research interests include deep learning and its application in robotic vision. Previously, Huangying received his B.Eng degree in Electronic Engineering (first class honors) from The Chinese University of Hong Kong (CUHK), where he was advised by Prof. Xiaogang Wang. Also, Huangying was a visiting student in the Unmanned Systems Research Group at The National University of Singapore, where he worked with Professor Ben M. Chen.
Lachlan Nicholson
Queensland University of Technology
Lachlan graduated from QUT in 2016 with First Class Honours in a Bachelor of Electrical Engineering. Whilst completing his degree, he was appointed by the Centre to continue the mechanical and software upgrade of the SummitXL mobile robot as a summer research task. He also worked with the ACRV to complete his undergraduate thesis with a focus on Navigation, Object Detection, and Mobile Manipulation within an office environment. With a team from the Centre of Excellence he competed in the Amazon Picking Challenge of 2016, achieving 6th place in the final demonstration held in Leipzig, Germany. Lachlan is currently pursuing his PhD with the centre and his research is focused on Scene Understanding via Deep Learning, Semantics and SLAM.
Mina Henein
Australian National University
Mina joined ANU and the Centre in March 2016 as a PhD candidate to work on SLAM in dynamic environments. He is doing research under the supervision of Viorela Ila and Robert Mahony. His research interests include graph-based SLAM, dynamic SLAM and object SLAM besides kinematics and optimization techniques.
Mina received a B.Sc. in Engineering and Materials Science with Honours majoring in Mechatronics from the German University in Cairo (GUC), Egypt in 2012. He then worked in the business sector for a multinational FMCG for one year as a Near-East demand manager before pursuing his masters in Advanced Robotics. He received a double M.Sc. degree; European Masters of Advanced Robotics (EMARo) from Universita degli Studi di Genova, Italy and Ecole Centrale de Nantes, France. Mina completed his PhD in 2020 and is now working as a research fellow at The 3Ai institute at ANU.
Jun Zhang
Australian National University
Jun is a PhD researcher in the Centre at ANU. He received both his B.Eng. and M.Sc.Eng. degrees at Northwestern Polytechnical University. During his master’s period, Jun spent one and half years in Peking University as a visiting researcher. His research interests lie in the area of robotic vision, particularly in visual odometry/SLAM, structure from motion, 3D vision and motion field.
Mehdi Hosseinzadeh
University of Adelaide
Mehdi was a PhD researcher in computer vision at the University of Adelaide under the supervision of Chief Investigators Ian Reid and Anton van den Hengel. He obtained his bachelor degree in Electrical Engineering in 2009 and his masters degree in Control Systems in 2013. His research interests are semantic visual SLAM, probabilistic graphical models and machine learning in robotic vision applications.
Mehdi completed in PhD in 2019.
Sourav Garg
Queensland University of Technology
Sourav is a robotic-vision enthusiast. His research spans computer vision, robotics and deep learning, motivated by practical applications that involve a moving camera. He pioneered research in the twin challenge of visual place recognition that requires dealing with scene appearance and camera viewpoint simultaneously. His award-winning research and PhD thesis proposed novel ways of robot localization based on visual semantics, inspired by humans. He is always keen on exploring research problems related to scene understanding and robot navigation, particularly those revolving around effective representation and matching of visual information.
Currently appointed as Postdoctoral Research Fellow with the QUT Centre for Robotics (QCR), Sourav’s past research experience includes working with various government organisations, conducting collaborative research involving several educational institutes, handling various robotics setups: indoor robots for office and retail spaces, drones for delivery and autonomous cars fitted with a suite of sensors. He received his PhD from QUT in 2019 while being a part of the Centre and is currently associated with the Centre as a Research Affiliate.
Shin Fang Ch’ng
University of Adelaide
Shin joined the Centre as a PhD researcher in 2017 under the supervision of Tat-Jun Chin and Yasir Latif. She graduated from Sheffield Hallam University, UK with first class honours in Electronics Engineering in 2012. Shin’s research interest lies in computer vision and robotics.
Jiawang Bian
University of Adelaide
Jiawang is currently a PhD researcher at the University of Adelaide and an Associated PhD researcher with the Centre. He is advised by Professor Ian Reid and Professor Chunhua Shen. His research interests lie in the field of computer vision, machine learning, and robotics. Jiawang received his B.Eng degree from Nankai University, where he was advised by Professor Ming-Ming Cheng. He was a research assistant at the Singapore University of Technology and Design (SUTD), where he worked with Professor Sai-Kit Yeung. Jiawang also worked as a trainee research engineer at the Advanced Digital Sciences Center in Singapore (ADSC), Huawei Technologies Co., Ltd, and Tusimple.
Anh-Dzung Doan
University of Adelaide
Dzung is a PhD candidate at the Australian Institute for Machine Learning in Adelaide, under the guidance of Professor Tat-Jun Chin, Dr. Yasir Latif, and Professor Ian Reid. Before that, he spent 3 years at Temasek Labs@SUTD in Singapore as a full-time research assistant, advised by Associate Professor Ngai-Man Cheung. He received Bachelor of Science with Honours (in top 1%) at the Vietnam National University – Ho Chi Minh City (University of Science).
His research interest is robotic vision, at the intersection of robotics, computer vision, and machine learning. For his PhD research, his focus is on visual place recognition under appearance changes for autonomous driving. After more than 5 years of conducting research in both academic and industrial sectors, he acquires an extensive expertise in developing scalable algorithms for visual place recognition, visual localization, and image retrieval.
Project Aim
As a robot moves around it needs to develop an understanding of its environment – the geometry, the objects present, the free-space, and the potential ways it can interact with objects and other elements of the environment (affordances).
This research project endowed robots with the ability to visually sense the geometry of a potentially uncertain and changing environment and to recognise objects and regions so that robot can build a high-level, dynamic map of the world. The ability to form this kind of high-level representation of the world is fundamental to a robot reasoning about a scene so that it can plan actions effectively and interact with its environment.
The project combined work on mapping an environment geometrically with work on understanding images and video in terms of their constituent semantic parts – the objects and regions in the scene. This has been tackled within the project in two main ways: developing new methods for performing visual localisation and mapping (building a map of the environment and working out where the robot is within that environment); and developing methods that leverage the power of deep learning to understand images and video.
Key Results
Research Fellow Yasir Latif and PhD Researcher Anh-Dzung Doan continued work on scaling their place recognition system, which was presented at the 2020 International Conference on Robotics and Automation (ICRA), to huge datasets. This has involved implementing memory management mechanisms and building data collection tools to harvest from mapillary (a crowd sourced street view and map database), and resulted in publications in Robotics and Automation Letters and Neural Computing Applications.
PhD researchers Mina Henein and Jun Zhang produced a system for Dynamic SLAM published at ICRA 2020. At this same venue PhD Researcher Huangying Zhan presented his state-of-the-art visual odometry research (how far and fast has the robot moved). In this work he has shown how to combine self-supervised deep learning for image matching with model selection. He and fellow PhD researcher Jiawang Bian continued to push the boundaries of self-supervised monocular visual odometry and depth estimation, showing that a number of simple but very effective ideas such as pre-rectification of images can be very helpful for learning depth in indoor scenes.
PhD Researcher Kejie Li collaborated with researchers at University College London and Facebook Realty Labs to create an object-based mapping system FroDo: From Objects to Detections, published at the 2020 Computer Vision and Pattern Recognition (CVPR) conference. This work combined earlier outputs from his PhD on single-view object reconstruction with a mapping system and more detailed (but slower) implicit function deep representations of objects to allow for either rapid coarse reconstruction, or slower but fine-grained object reconstruction within the overall scene reconstruction. His follow-up work which includes the presence of dynamic objects and improved data association has been accepted for Robotics and Automation Letters (and ICRA 2021).
Research Fellows Pulak Purkait and Ravi Garg continued the Centre’s work on using deep learning for solving geometric tasks. In particular Purkait first-authored two papers at the 2020 European Conference on Computer Vision (ECCV). The first showed how to learn a compact scene grammar using a deep autoencoder, and to use this to improve indoor high-level (object-based) scene reconstruction. The second, NeuRoRa, demonstrated the power of deep learning within optimisation problems, showing how a learned approach to rotation averaging in large-scale reconstruction problems yields better convergence and improved accuracy.
In 2020 we consolidated significant outcomes from the project into our open-source repository ‘Best of ACRV’. In particular: PhD Researcher Lachlan Nicholson converted project outcome QuadricSLAM; PhD researchers Huanying Zhan and Ah-Dung Doan worked with postdocs Yasir Latif and Wei Liu to create a system for topological mapping by combining their respective state-of-the-art visual odometry and place recognition systems. In work led by PhD researcher Sourav Garg, we also consolidated our work on semantics for robotic mapping into a seminal survey paper, published in Now Foundations and Trends.
Feature image photo credit: Artur Debat, Moment, Getty Images